Precision Agriculture: Navigating Climate Change Challenges for Sustainable & Profitable Farming
Alexandre Croteau and Alicia Guay
Introduction
Rising temperatures, altered precipitation patterns, and increased extreme weather events due to climate change pose significant threats to agriculture by impacting crop yields, water resources, and overall sustainability (Intergovernmental Panel on Climate Change [IPCC], 2022). This scenario complicates global food security efforts and stresses agricultural systems. The situation is aggravated by the projected 50% increase in food production demand by 2050, driven by population growth and the rising need for biofuels (Medel-Jiménez et al., 2023). GHG emissions from agricultural production emitted 9.3 Gt CO2eq in 2018 (Food and Agriculture Organization of the United Nations [FAO], 2020). Against this backdrop, transformative precision agriculture (PA) technologies, including variable-rate technology (VRT), guidance systems (GS), and geographic information system (GPS) mapping, as well as other emerging technologies such as artificial intelligence (AI) and remote sensing (RS), offer viable solutions to climate change challenges. This commentary explores the economic implications of these technologies globally and in the Canadian context.
Precision Agriculture
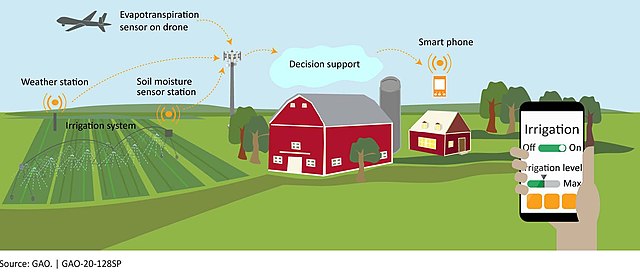
Economic Implications
PA uses GPS, sensors, and data analytics to enhance farming practices, boosting productivity, reducing environmental impacts, and mitigating GHG emissions (Balfoutis et al., 2017). These technologies enhance management practices, reduce costs, and improve yield outcomes, presenting substantial economic benefits for the agricultural sector. A study published by Schimmelpfenning (2016) found that adopting PA technologies could lead to a noticeable increase in profit margins for farmers, although the magnitude of benefits could vary by crop and technology. According to the summary report of Schimmelpfenning (2016), the adoption of PA technologies has a small positive impact on both net returns (including overhead expenses) and operating profits for a U.S. corn farm of average size (see Figure 2). Specifically, GPS mapping shows the largest estimated impact among PA technologies, with an increase in operating profit of almost 3% on corn farms.
Beyond individual profit margins, PA contributes to broader economic benefits by enhancing sustainability, reducing environmental impacts through more efficient use of inputs, and contributing to food security by increasing production efficiency (Nicol & Nicol, 2018). However, the initial investment in PA can be significant. This investment includes the cost of hardware (GPS devices, sensors, drones and automated machinery) and software (data analysis and management).
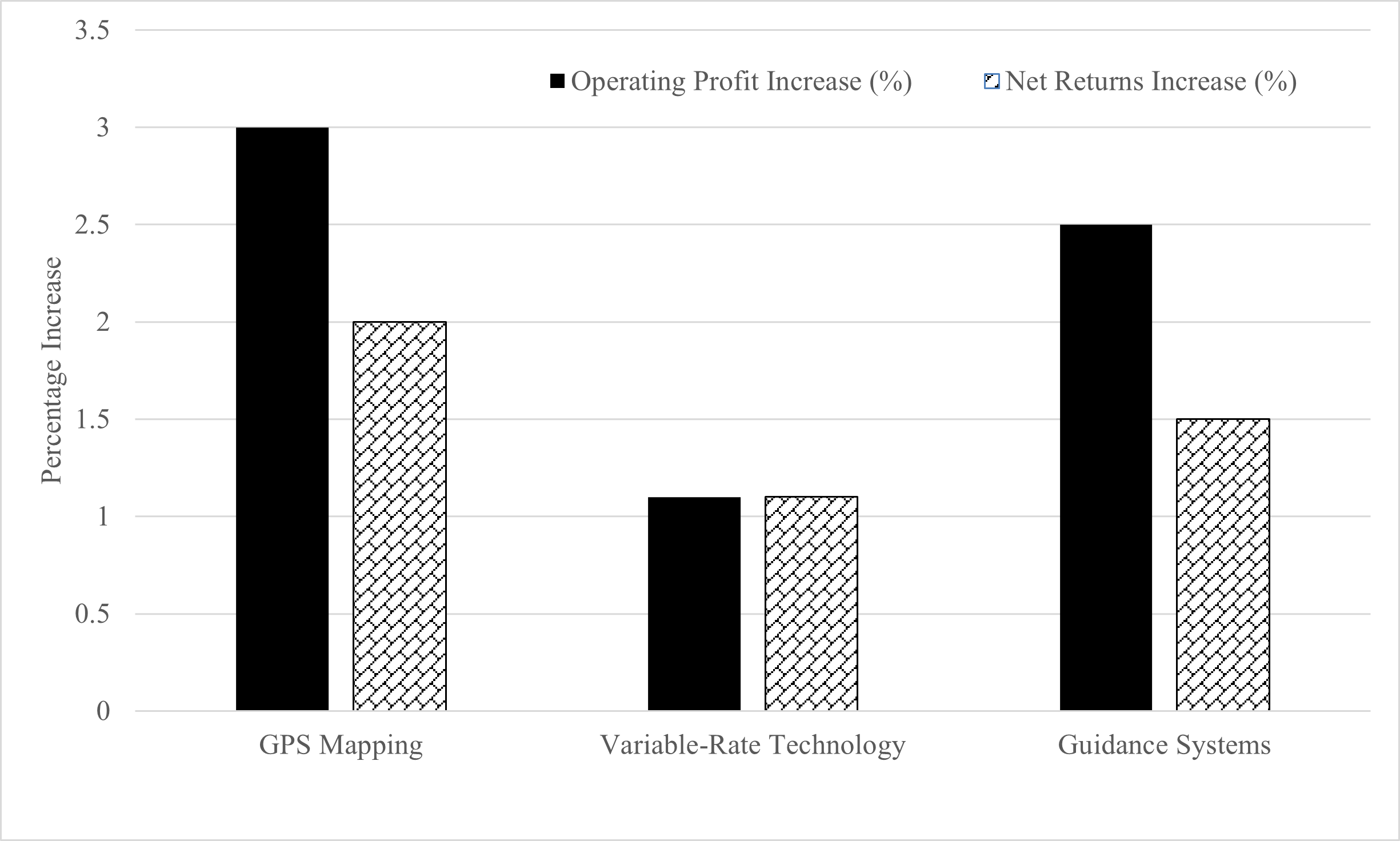
Canadian Agricultural Landscape
In 2022, Canada’s agricultural sector, which contributed 8.1% to the country’s total GHG emissions, employed 249,900 people and generated $36.3 billion, accounting for approximately 1.8% of the GDP (Environment and Climate Change Canada, 2023; Agriculture and Agri-Food Canada, 2023). The 2021 Census of Agriculture in Canada highlights the growing adoption of PA technologies, such as GIS mapping, slow-release fertilizers, and drones, indicating a shift toward more efficient and sustainable practices. For instance, GIS mapping increased on Canadian farms from 8.2% in 2015 to 13.2% in 2020, allowing for detailed crop cultivation data collection and optimized yield planning (Chen & Jewitt, 2023).
Other Transformative Technologies
The AI agriculture market, which stood at $1,254.6 million, is projected to expand by 26.7% from 2022 to 2030, reaching $8,308.5 million (Sakapaji & Puthenkalam, 2023). AI improves crop management, reduces pest and disease losses, optimizes resource use, and increases yields (Ampatzidis, 2018; Ennouri et al., 2021). Meanwhile, RS technology facilitates efficient crop health and environmental condition monitoring, enabling timely risk mitigation (Ennouri et al., 2021; Vidican et al., 2023).
Challenges to Adoption & Policy Recommendations
Despite their benefits, adopting these technologies faces challenges, including high initial costs, a lack of technical expertise, and data privacy concerns (Mitchell et al., 2020; Nicol & Nicol, 2018; Sakapaji & Puthenkalam, 2023). Government policies and initiatives, such as subsidies, tax incentives, and investments in research and development, are essential to facilitate adoption. These measures can help overcome adoption barriers, paving the way for a more sustainable and productive agricultural future.
Media Attributions
Figure 1: “Figure 16: Components of a Precision Agriculture System” by the U.S Government of Accountability Office (2019) is used under the U.S. Government of Accountability Office Terms of Use.
Figure 2: “Comparative impact of precision agriculture technologies on U.S. Corn farms” was created by the authors under a CC BY-NC-SA 4.0.
References
Ampatzidis, Y. (2018). Applications of artificial intelligence for precision agriculture: AE529, 12/2018. EDIS, 2018(6). https://doi.org/10.32473/edis-ae529-2018.
Balafoutis, A., Back, B., Fountas, S., Vengeyte, J., der Wal, T. V., Soto, I., Gómez-Barbero, M., Barnes, A., & Eory, V. (2017). Precision agriculture technologies positively contributing to GHG emissions mitigation, farm productivity and economics. Sustainability, 9(8), Article 1339. https://doi.org/10.3390/su9081339.
Chen, Z. J., & Jewitt, A. (2023, May 17). Canada’s farms integrate renewable energy production and technologies toward a future of sustainable and efficient agriculture. Statistics Canada, Government of Canada. https://www150.statcan.gc.ca/n1/pub/96-325-x/2021001/article/00016-eng.htm.
Ennouri, K., Smaoui, S., Gharbi, Y., Cheffi, M., Braiek, O. B., Ennouri, M., & Triki, M. A. (2021). Usage of artificial intelligence and remote sensing as efficient devices to increase agricultural system yields. Journal of Food Quality, 2021, Article 6242288. https://doi.org/10.1155/2021/6242288.
Environment and Climate Change Canada. (2023, April 14). Greenhouse gas sources and sinks in Canada: executive summary 2023. Government of Canada. https://www.canada.ca/en/environment-climate-change/services/climate-change/greenhouse-gas-emissions/sources-sinks-executive-summary-2023.html.
Food and Agriculture Organization of the United Nations. (2020). Emissions due to agriculture: Global, regional and country trends 2000–2018 (FAOSTAT Analytical Brief 18). https://www.fao.org/3/cb3808en/cb3808en.pdf.
Intergovernmental Panel on Climate Change. (2022). Climate change 2022: Impacts, adaptation and vulnerability (Contribution of Working Group II to the Sixth Assessment Report of the Intergovernmental Panel on Climate Change). Cambridge University Press. https://doi.org/10.1017/9781009325844.
Medel-Jiménez, F., Krexner, T., Gronauer, A., & Kral, I. (2024). Life cycle assessment of four different precision agriculture technologies and comparison with a conventional scheme. Journal of Cleaner Production, 434, Article 140198. https://doi.org/10.1016/j.jclepro.2023.140198.
Mitchell, S., Weersink, A., & Bannon, N. (2020). Adoption barriers for precision agriculture technologies in Canadian crop production. Canadian Journal of Plant Science, 101(3), 412–416. https://doi.org/10.1139/cjps-2020-0234.
Nicol, L. A., & Nicol, C. J. (2018). Adoption of precision agriculture to reduce inputs, enhance sustainability and increase food production: A study of southern Alberta, Canada. WIT Transactions on Ecology and the Environment, 217, 327–336. https://doi.org/10.2495/sdp180301.
Sakapaji, S. C., & Puthenkalam, J. J. (2023). Harnessing AI for climate-resilient agriculture: opportunities and challenges. European Journal of Theoretical and Applied Sciences, 1(6), 1144–1158. https://doi.org/10.59324/ejtas.2023.1(6).111.
Schimmelpfennig, D. (2016, October). Farm profits and adoption of precision agriculture (Report No. ERR-217). United States Department of Agriculture. https://www.ers.usda.gov/publications/pub-details/?pubid=80325.
U.S Government of Accountability Office. (2019). Figure 16: Components of precision agriculture system [Image from Report No. GAO-20-128P]. https://www.gao.gov/products/gao-20-128sp.
Vidican, R.; Mălinaș, A.; Ranta, O.; Moldovan, C.; Marian, O.; Ghețe, A.; Ghișe, C.R.; Popovici, F.; Cătunescu, G.M. (2023). Using Remote Sensing Vegetation Indices for the Discrimination and Monitoring of Agricultural Crops: A Critical Review. Agronomy, 13, 3040. https://doi.org/10.3390/agronomy13123040